NCS Journal Club Round-Up #NCSTJC: August 2023
Published on: December 19, 2023
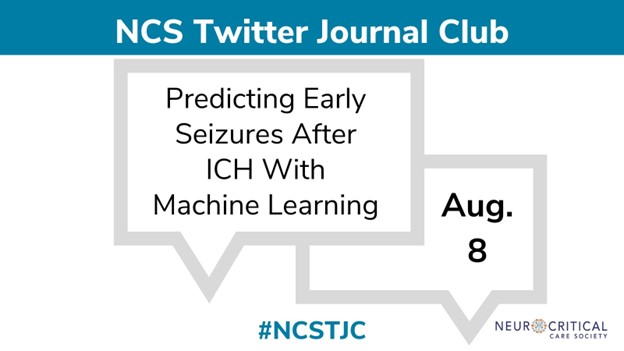
Moderators: @KeatonSmetana
Inspired by the opening keynote address of the Neurocritical Care Society Annual Meeting, the August Neurocritical Care Society Twitter Journal Club featured the article "Predicting Early Seizures After Intracerebral Hemorrhage with Machine Learning" by Gabrielle Bunney et al. The article was an original research study on the development and validation of machine learning models to predict early seizures after ICH. The authors utilized two datasets to train their machine-learning model and validated it with a separate data set.
It was fitting that this discussion on machine learning took place in the electronic realm of Twitter. We will review the discussion topics, polls answered, and opinions expressed below.
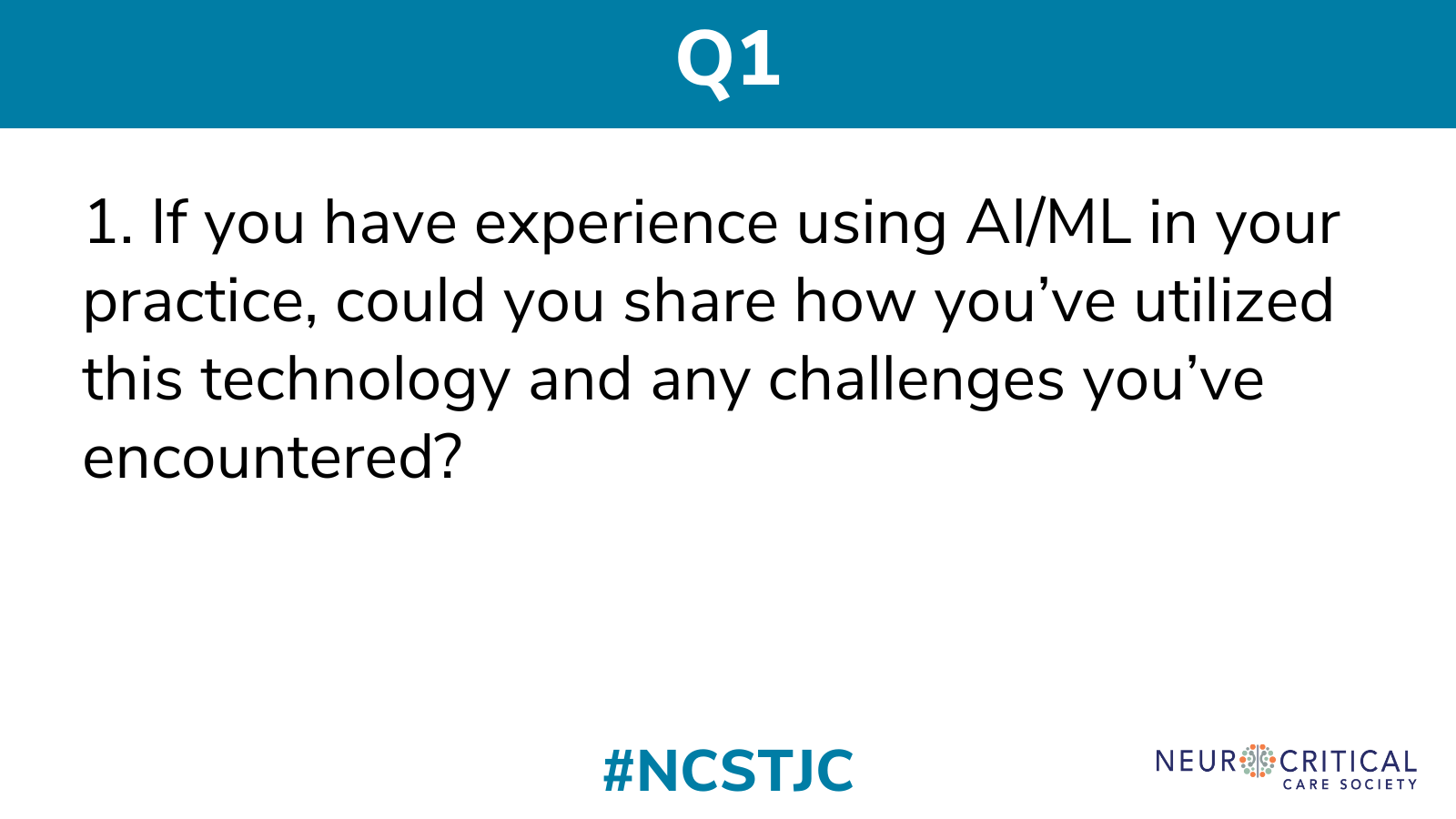
The first question asked participants to consider their experience with artificial intelligence (AI) and Machine Learning (ML) and share any challenges encountered. First author Dr. Gabrielle Bunney provided her personal experience stating, “Working on effectively translating these models into the clinical space is incredibly challenging.” She went on to discuss the difficulties of integrating ML models into the electronic health record and remarked, “Extensive iteration and further study is incredibly important to move beyond models in literature to models in practice.” @Gabeknowz joined the discussion stating, “@RapidAI, @viz_ai, and others have single-handedly revolutionized acute stroke care in the #USA. Future technologies should aim to mirror their implementation in acute stroke triage algorithms in other fields.”
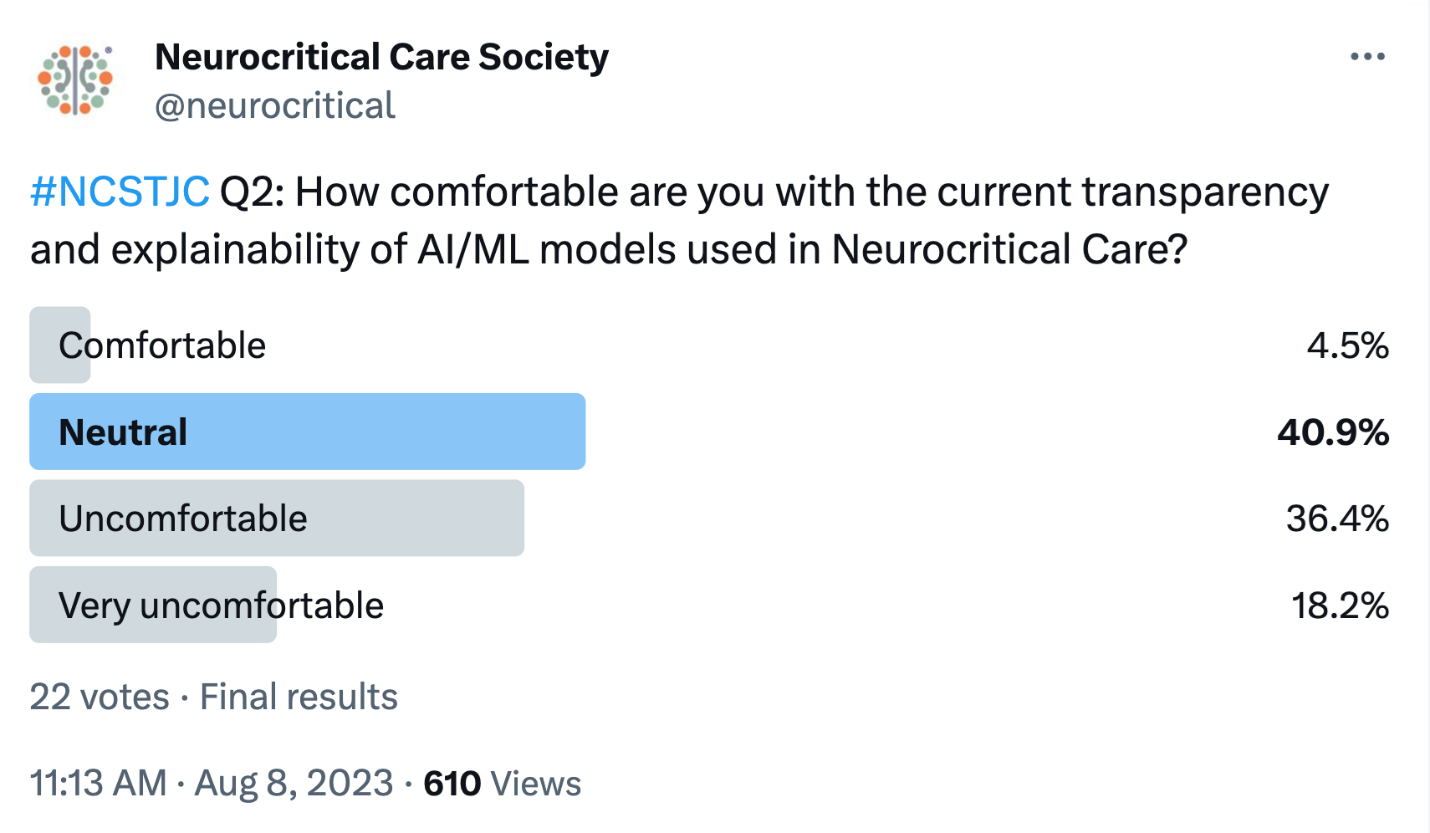
Question two was in the form of a poll and asked participants their comfort level on the transparency and explainability of AI and ML models used in neurocritical care. Only a very small portion (4.5%) of respondents indicated they were comfortable with the current state of the models. All other respondents were split between neutral and uncomfortable or very uncomfortable.
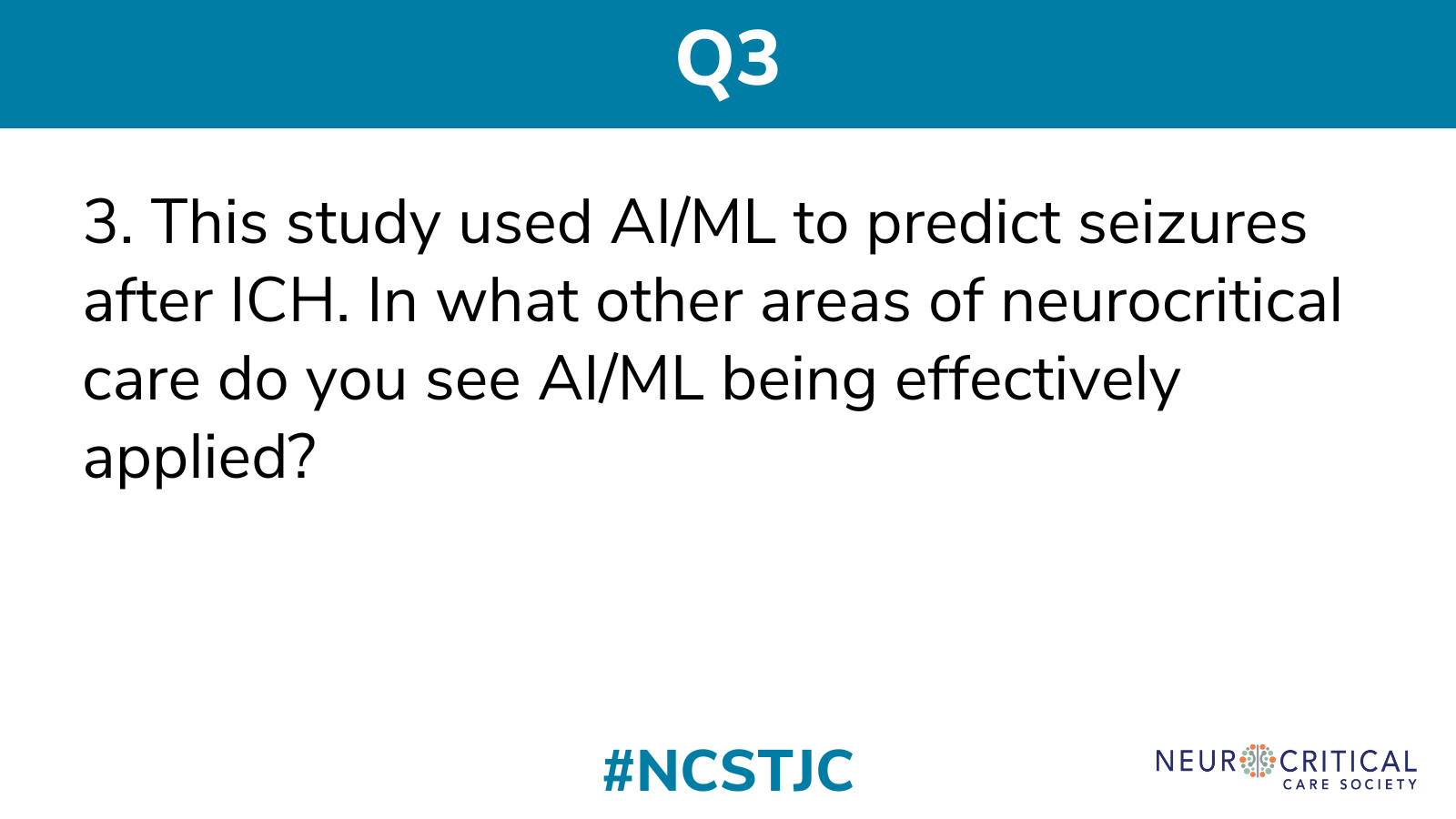
Question three is still open for debate, as participants were left without words to answer what other areas AI/ML might be effectively applied within neurocritical care. Based on the answers to question 2, it would seem most want to gain a better understanding and level of comfort with AI/ML before expanding it further into the field.
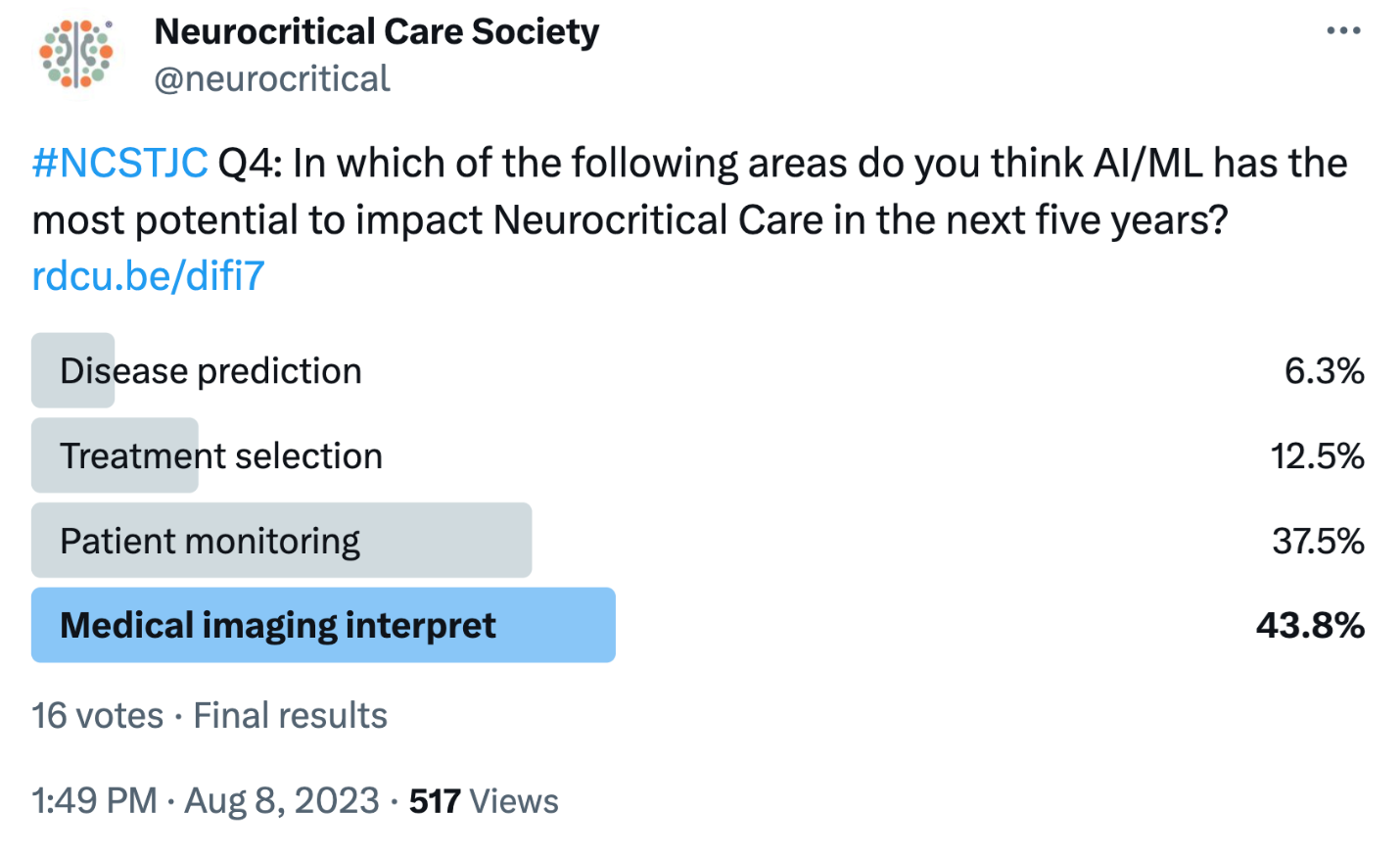
Question four gave respondents a more direct way to indicate where they thought AI/ML might expand within the field of neurocritical care. Most indicated that medical imaging interpretation was the part of the field most ripe for impact in the near future. However, patient monitoring and treatment selection were also frequent answers. Very few (6.3%) thought it could be used for disease prediction, which is interesting considering the authors’ conclusion in this study showing the utility of their models in predicting seizures in ICH.
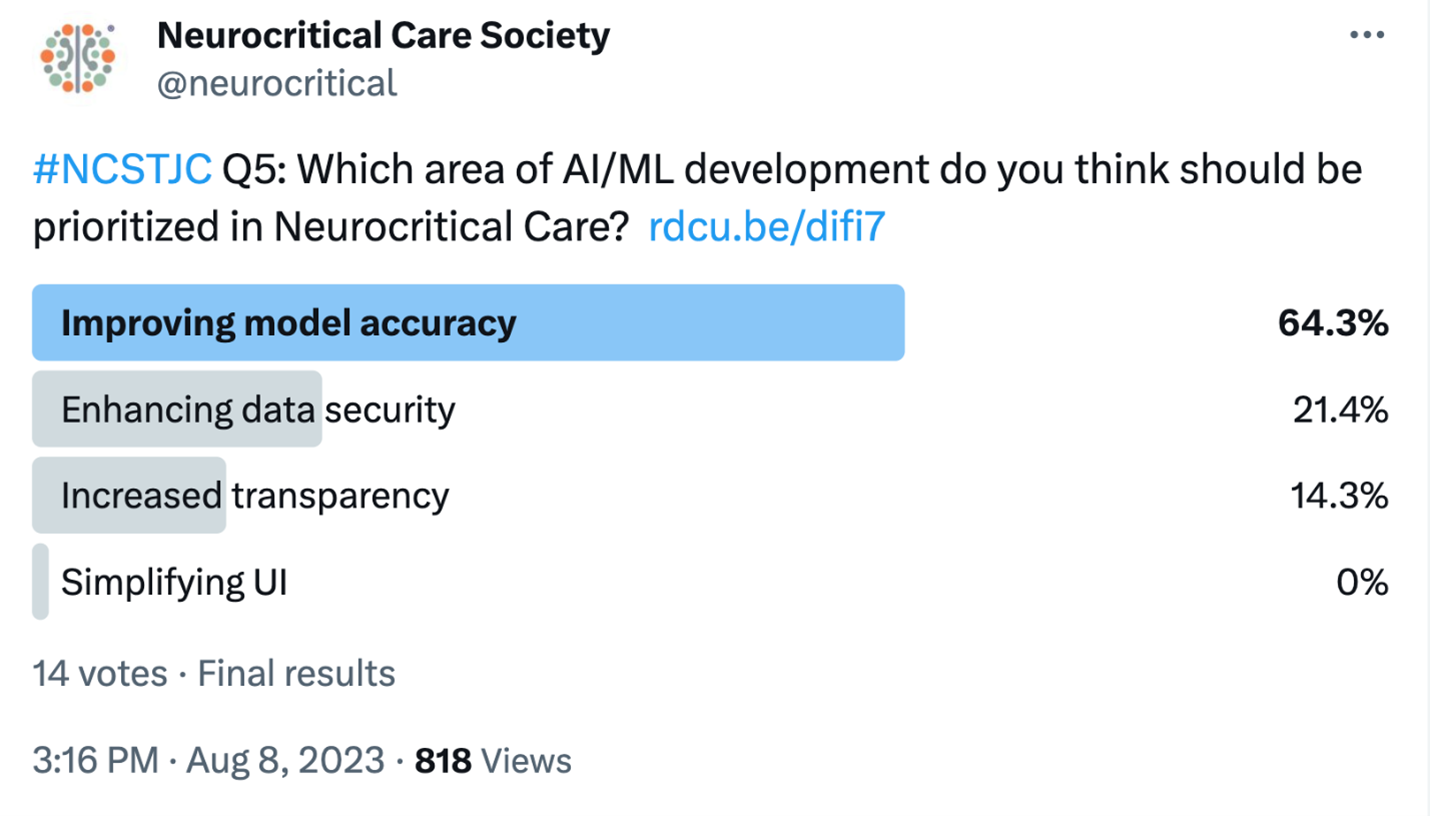
Fitting with the skepticism that came across in answers to earlier questions, participants felt that improving model accuracy should be the highest priority for the use of AI/ML in neurocritical care. So while we can discuss potential avenues for expansion, overall participants felt that building on and improving what we know in AI/ML should be the top priority. There were also a few respondents who felt enhancing data security and increased transparency would be priorities. Dr. Gabrielle Bunney, one of the authors of the study, contributed by stating, “I believe that all of those are large barriers to wider adoption of AI/ML, but having a standardized way in which we validate models is incredibly important.”
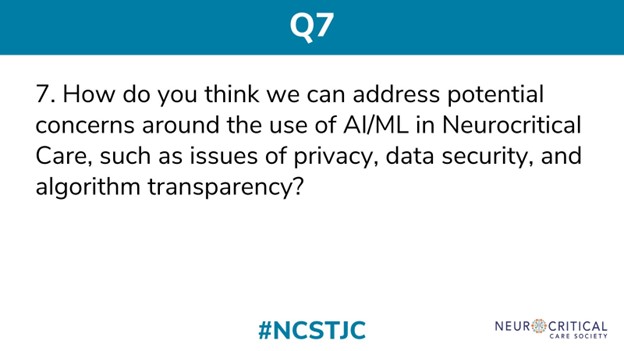
We skipped over question six and ended with a final question asking participants to pitch their ideas for improving privacy, data security, and transparency in AI/ML, specifically within neurocritical care. Dr. Gabrielle Bunney provided her thoughts that “Privacy and security issues are likely going to require regulatory involvement. Algorithm transparency comes inherently from the algorithm itself. As the research into the technical aspects of the models themselves improve, so will the explainability.”
***
The August edition of the #NCSTJC followed the theme of the keynote of the NCS Annual Meeting highlighting the use of AI and ML in neurocritical care. The online polls indicated a continued healthy skepticism of ML models and AI as we think about its translation to clinical care. Most cited their level of discomfort resides in a lack of transparency of the models, combined with a need for further and more uniform validation. The authors of the study concluded they were able to find early seizures after ICH could be predicted from routine clinical data. They felt the next step is to utilize the ML model in a clinical trial to ultimately help predict which patients would benefit from prophylactic seizure medications.
References
Bunney, G., Murphy, J., Colton, K. et al. Predicting Early Seizures After Intracerebral Hemorrhage with Machine Learning. Neurocrit Care 37 (Suppl 2), 322–327 (2022). https://doi.org/10.1007/s12028-022-01470-x